Dealing with true demand today to help customers for the coming months
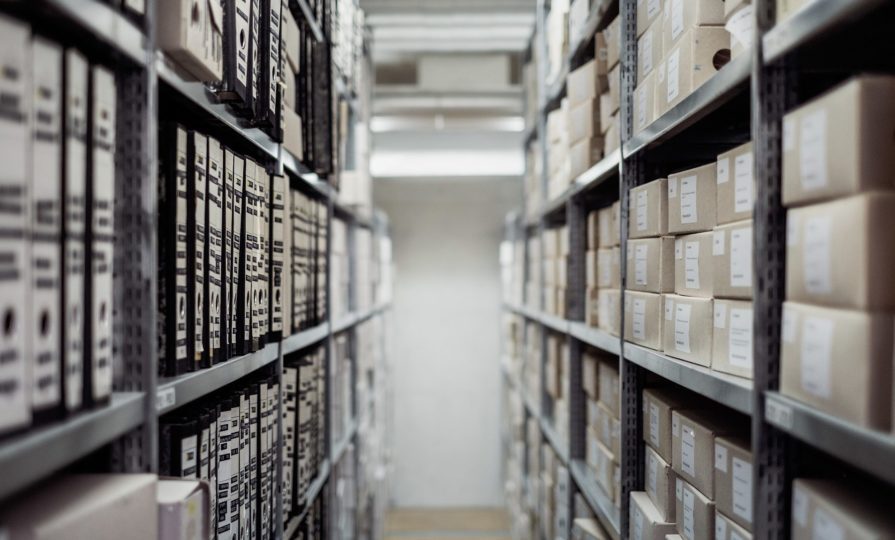
With the COVID-19 novel coronavirus on the rise worldwide, retailers are being forced to rethink their supply strategies on the fly. Demand for sundries and medical essentials is booming – especially via “buy online, pick up in store” (BOPIS) as well as curbside and home delivery channels that aid with social distancing.